Showing top 0 results 0 results found
Showing top 0 results 0 results found
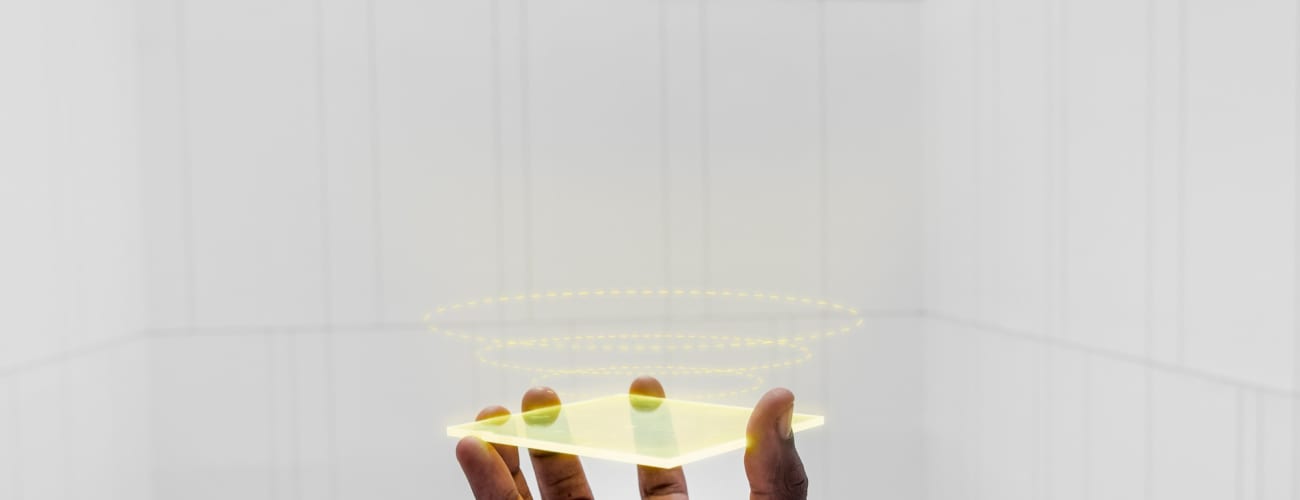
Providing an exceptional customer experience is critical for companies to succeed in the current business landscape. And keeping clients is more financially advantageous compared to obtaining new ones.
Artificial intelligence is becoming increasingly crucial in revolutionizing the customer service process. The technology offers many potential benefits, including improved customer service and increased efficiency.
What is AI customer experience?
Artificial intelligence (AI) customer experience involves harnessing the power of AI technologies to augment customer interactions, streamline business operations, and ultimately bolster overall customer satisfaction.
The technologies deployed in this approach range from machine learning (ML) algorithms to natural language processing (NLP), predictive analytics, and even robotic process automation. AI facilitates a highly personalized customer experience by sifting through large volumes of customer data, including browsing patterns, purchase history, and social media behavior.
This capacity for in-depth analysis enables businesses to discern individual customer preferences, thus tailoring their recommendations and interactions to cater to these specific tastes and needs.
AI's role in personalization and proactive issue resolution
Innovations such as artificial intelligence have made it possible to personalize customer experiences in various channels, including contact centers, brick-and-mortar stores, and interactive voice response systems, not just limited to marketing functions. This has opened up the opportunity to enhance the overall customer journey from beginning to end.
Personalization based on customer preferences can lead to remarkable outcomes by reducing customer churn and increasing customer lifetime value. By demonstrating empathy and concern for customers, personalizing the entire customer journey can provide more than just sales-related interactions and allow for customized responses to each customer's unique needs.
How to use AI in customer service
How can you use AI to make the best customer service possible? There are several ways; let’s cover the main ones.
Chatbots
Chatbots powered by AI are highly effective tools for modern customer service. They come equipped with NLP with machine learning algorithms, allowing them to understand and respond to customers in real time.
These AI-driven chatbots are different from traditional scripted chatbots because they can handle a broad range of queries using a more conversational approach similar to how humans communicate. They can assist users with various tasks, including answering basic questions, guiding them through website navigation, and troubleshooting common issues.
For example, a telecom company can deploy an AI chatbot on its website and mobile app. Suppose a customer visits the website looking to upgrade their plan. In that case, the chatbot can initiate a conversation with them, understand their request through natural language processing, and then guide them through the available options, helping them choose and activate a new plan – all without human intervention.
Chatbot tip
Integrating an AI chatbot properly with your existing customer service database and CRM systems is essential to ensure efficient functionality. This integration enables the chatbot to access relevant customer data, providing more personalized and accurate responses to customer inquiries. Furthermore, regularly updating and training your AI models with new data and customer interaction scenarios can help improve the chatbot's performance over time.
AI analytics
AI-powered predictive customer service tools can analyze large amounts of data and anticipate customer needs or problems before they occur. With data analytics and machine learning algorithms, companies can observe trends in customer behavior. This proactive approach enables businesses to tackle potential issues, provide timely solutions, or customize their services and products to cater to predicted needs. There are various advantages to utilizing AI customer support software.
AI analytics tip
To effectively implement predictive customer service, focus on collecting and analyzing a wide range of data points – from transaction history and customer service interactions to social media feedback.
The richer the data, the more accurate the predictions. Ensure compliance with data protection regulations to maintain customer trust. Regularly revisiting and refining your predictive models is key to keeping them relevant and effective in changing market conditions.
Self-service portals
AI-powered self-service portals are designed to help customers find answers and solve their problems independently, without the need to speak with a customer service representative. These portals leverage machine learning and natural language processing to learn how to respond to customer queries effectively.
They offer several features, such as intelligent search bars, personalized FAQs, and interactive guides, which are continuously updated based on user feedback and interactions.
For instance, a software company can incorporate an AI-powered self-service portal with an intelligent search function. When a user types in a query, the portal will provide relevant documentation, articles, and tutorials based on the user's past queries and product usage. If a customer frequently searches for topics related to a specific software feature, the portal may proactively suggest advanced guides or tutorials for that feature.
Self-service portals tip
Prioritize the user experience when developing an AI-enhanced self-service portal. It should be easy to navigate and have an intuitive interface. You can use customer interaction data to improve the portal constantly constantly.
If there are a lot of customer searches for a particular issue, consider creating a dedicated resource or guide for that topic. Additionally, ensure there is an easy option for customers to escalate their query to a human agent if the self-service portal cannot resolve their issue.
Customer feedback
AI-powered sentiment analysis utilizes NLP and machine learning to assess and comprehend the emotional tone underlying customer conversations, feedback, interactions, and reviews.
This technology empowers businesses to understand the sentiment of their customers, whether it is positive, negative, or neutral, and take appropriate action. By scrutinizing textual content from different sources such as social media, emails, and customer reviews, companies can acquire valuable insights into customer satisfaction, brand perception, and other areas requiring attention.
For example, a restaurant chain could leverage sentiment analysis to keep track of customer reviews and social media mentions. The AI system would detect a recurring pattern of negative sentiment regarding wait times at multiple locations.
The management can take specific measures to address the issue using this information. Like adjusting staffing levels or enhancing processes, thereby directly tackling customer concerns.
Customer feedback tip
When implementing sentiment analysis, it’s essential to consider the nuances and context of language. AI models should be trained on a diverse dataset to understand different expressions, slang, and regional language variations.
Additionally, integrate sentiment analysis with your customer relationship management (CRM) system for a holistic view. Use these insights to guide business strategies and customer service policies.
AI customer support software for specific industries
AI customer support can be used in many different industries. Here are some examples:.
Insurance
Insurance companies often suffer significant yearly financial losses due to their vulnerability to fraud. Fortunately, integrating AI within the insurance industry has improved the capabilities of detecting and preventing fraud. With deep learning technologies and machine learning, AI solutions can detect unusual customer behavior patterns that may indicate fraudulent activity. These systems also support conducting thorough background checks of potential customers before onboarding, ultimately improving customer service quality.
Telecommunications
Automated technologies can aid in the streamlining of call center operations by reducing the occurrence of manual errors and performing repetitive tasks. These technologies can include self-serve systems, conversational IVR, which can understand customer voice commands, and agent assist tools that provide real-time guidance to agents during calls.
Banking
Advanced technologies such as sentiment analysis and emotion recognition powered by artificial intelligence are employed to analyze customer interactions to unravel underlying emotions.
This helps banks to get a better understanding of their customers and provide more tailored experiences while being proactive in addressing any issues that may arise. Bank of America leverages this technology to carefully scrutinize customer feedback andto pinpoint improvement areas for improvementcarefully.
Retail and ecommerence
AI-powered systems analyze customer data to create customized product recommendations. This technology helps e-commerce businesses improve user experience by suggesting products that match customers' buying history and preferences. For instance, Amazon uses AI to recommend products based on a user's browsing and purchasing behavior.
Healthcare
AI can improve appointment management by making it more efficient, which can help reduce wait times and improve patient satisfaction. With the help of an AI assistant, appointments can be scheduled, rescheduled, or canceled seamlessly. Moreover, the AI assistant can provide reminders, specify the required documentation, and give detailed instructions for appointment preparation.
Supply chain and logistics
Businesses that handle a considerable volume of inventory can benefit from using AI in inventory management. By utilizing an AI system, companies can monitor and regulate inventory levels in real timereal-time, thus preventing shortages or overstock. This can help businesses save significant money and avoid losing sales due to insufficient stock.
Manufacturing
AI-powered chatbots have the potential to keep all stakeholders, including employees and customers, informed about delivery updates. These chatbots can provide up-to-date information about delivery status, timing, and condition, saving customer support teams valuable time and improving customer satisfaction.
Manufacturing companies can benefit from AI chatbots that provide product recommendations. Using machine learning algorithms and historical data, these chatbots can understand customer preferences and suggest suitable products to enhance the buying experience.
How AI optimizes resources
Ai optimizes resources by taking on the grunt work and letting humans handle more important matters. There are several ways AI can help with optimizing resources.
Demand forecastingforcasting
Demand forecasting is an important resource optimization in e-commerce. AI, equipped with machine learning models, can analyze customer behaviors, seasonal trends, and a myriad of data touchpoints to provide uncannily accurate demand predictions. This proactive approach ensures businesses don't overstock or understock, ultimately maximizing resources.
Intelligent inventory management
AI doesn't just stop at forecasting; it extends into inventory management. Smart algorithms can quickly monitor stock levels, anticipate shortage risks, and recommend maintaining the right inventory. This data-driven precision results in minimized capital tied up in unsold stock or lost sales due to inadequate inventory.
Dynamic pricing strategies
Resource optimization isn't solely about what you have; it's about getting the most out of it. AI's real-time market analysis capabilities can mean that prices are neitherver too high to put off potential buyers nor too low to lose potential profit. By adjusting pricing dynamically, AI ensures businesses remain competitive while maximizing revenue—AI customer support solutions for small businesses and enterprises.
Personalized and automated marketing
Resource allocation in marketing can be a minefield without the right data. AI can personalize marketing messages and promotions at scale, ensuring that resources are invested where they will yield the highest return. The result? Targeted campaigns that perform better and cost less, freeing up resources for other business areas.
Efficient returns and refunds processing
Dealing with product returns and refunds is an inevitable part of e-commerce. AI can streamline this process by analyzing the reasons for returns and suggesting courses of action. This efficiency saves time and resources and provides insights to improve product quality or customer satisfaction—essential features of effective AI customer support software.
Healthier workforce management
Optimizing human resource management is possible with the help of AI. AI can predict peak times and arrange employee schedules accordingly, ensuring the correct number of workers is always present. This leads to increased efficiency and happier, more productive employees.
Advancements in chatbots
Chatbots have had significant advancements over the past few years and here are some of the most notable ones.
Natural Language Processing (NLP) breakthroughs
This section will discuss the improvements in NLP that that have helped improveimproved chatbots.
Enhanced understanding with NLP
Natural Language Processing (NLP) is at the heart of effective chatbot communication, transforming how bots interpret and respond to human language. Recent breakthroughs include advanced NLP models with unprecedented abilities to grasp the nuances of speech, allowing chatbots to process a broader spectrum of queries with higher precision.
Bidirectional encoder representations
Bidirectional eEncoder rRepresentations from tTransformers (BERT) have caused a paradigm shift in NLP. BERT-based chatbots can comprehend the full context of a conversation, recognizing relationships between words and the meaning behind users' intents. This breakthrough has dramatically improved chatbot accuracy and contextual understanding, enhancing the customer experience.
Transfer learning
Transfer lLearning allows chatbots to apply knowledge gained from one language or domain to another, significantly shortening bots' training time while maintaining or improving their comprehension in different scenarios. This approach has democratized the development of multilingual and multi-domain chatbots, making them more accessible for businesses of all sizes and global reach.
Machine Llearning (ML) for dynamic conversations
In this section, you’ll learn more abouton how ML has helped chatbots improve their natural language processing.
Adaptive models for personalized experiences
Machine lLearning empowers chatbots to deliver personalized interactions that adapt to user preferences and evolving conversation contexts. ML-powered chatbots learn from past interactions, continually enhancing their responses to ensure each conversation is more insightful and tailored to the individual's needs.
Conversational memory
Chatbots now possess a memory that goes beyond the current session. They can recall past interactions, understand user history, and maintain a coherent thread of conversation over time, mirroring the continuity that people expect in ongoing dialogues. This development has profound implications for relationship-building and customer retention.
Dynamic responses
With chatbots that use mMachine lLearning, the level of automation can be finely balanced against the value of human involvement. These bots can gauge user satisfaction in real -time and seamlessly transfer to a human operator, ensuring complex or critical queries receive the personalized attention they need without interrupting the flow of the conversation.
Multimodal interfaces and omnichannel support
Let’s look atHere you will learn more about how chatbots have multimodal interfaces and omnichannel support.
A unified front for customer engagement
Multimodal interfaces allow chatbots to interact through various channels and devices, from text and voice to images and videos. This capability and omnichannel support provide customers with a seamless experience across touchpoints, eliminating the frustration of repeating information when switching between platforms.
Voice recognition
Chatbots now boast advanced voice recognition technology that enables them to accurately understand spoken language. Integration with voice assistants and IVR systems has taken conversational interfaces to new heights, offering unparalleled convenience in scenarios where users' hands are tied.
Visual analytics and support
The Iintegration of visual analytics allows for more personalized and accurate customer support team together. For instance, a user could capture an image of a product and upload it to a chatbot channel for immediate troubleshooting, saving time and reducing errors in the issue descriptiondescription of the issue, enhancing communication efficiency and customer satisfaction.
Emotional intelligence and sentiment analysis
Chatbots are now getting more emotionally intelligent using sentiment analysis.
The art of empathy in AI
Understanding the emotional cues of customers has long been a challenge for chatbots. However, advancements in emotional intelligence and sentiment analysis advancements enable chatbots to recognize and respond to users' moods and attitudes, fostering more meaningful and empathetic exchanges.
Empathetic responses
Chatbots are now equipped with pre-built emotional models and language generation systems that enable them to respond tactfully and sensitively. These responses can acknowledge a customer's hardships or excitement, contributing to a more human-like and resonant interaction.
Sentiment-driven insights
By leveraging sentiment analysis, chatbots can deduce customers' emotional states from their language and use this insight to shape the conversation. For instance, a positive sentiment might trigger the bot to share promotional offers. At the same time, a negative one could prompt an apology and swift resolution to an issue, fine-tuning the customer experience in real time.
Continuous learning and ethical considerations
Despite the Chatbots continualcontinously improvement of chatbots, there arend several ethical considerations to that shouldthere are several ethical considerations that should be considered.
The journey towards digital maturity
As chatbots become more sophisticated, ethical considerations around their development and usage have gained prominence. Continuous learning mechanisms ensure that chatbots remain up-to-date with the latest data protection laws and ethical guidelines, incorporating these principles into their operations and interactions.
Transparent AI
Chatbots now include features that disclose when users speak with AI rather than a human agent. This transparency respects user privacy and sets clear expectations about the limitations and capabilities of the chatbot, building trust with customers.
Privacy-preserving technologies
Incorporating federated learning and differential privacy into chatbot training enhances data privacy, safeguarding sensitive customer information. By keeping data on the edge rather than the central server, businesses can assure their clientele that their personal information is protected while still benefiting from the insights gained through ML models.
Break down the pricing structure
The pricing architecture of AI customer service software is primarily predicated on subscription models, which are delineated into distinct tiers. These tiers are meticulously designed to cater to the heterogeneous requirements of businesses ranging from budding enterprises to large-scale organizations.
Basic tier
The foundational tier typically encompasses rudimentary features for initiating AI-driven customer service operations. It may include automated responses, a limited set of templates for customer interaction, and basic analytics for monitoring performance. The subscription cost for this tier is generally positioned to attract small businesses seeking to automate their customer service function without incurring substantial expenditure.
Intermediate tier
This tier represents an advanced subscription model that expands upon the Basic tier by incorporating more sophisticated features. Enhancements may incorporate more intricate AI models capable of understanding and processing natural language queries with greater insight, integration capabilities with other business systems (such as CRM software), and more comprehensive analytics. The Intermediate tier is designed for businesses with moderate customer service demands looking to leverage more advanced AI functionalities.
Premium tier
The zenith of the subscription models, the Premium tier offers the most exhaustive compilation of features. This may include bespoke AI solutions tailored to an organization's specific needs, omnichannel support facilitating seamless customer service across various platforms, and state-of-the-art analytics employing machine learning algorithms for predictive customer behavior analysis. The Premium tier is for large organizations with complex customer service requirements.
Additional costs and potential hidden fees
While the subscription tiers encapsulate the primary costs associated with AI customer service software, businesses must be aware of additional expenses that may ensue.
Customization fees
While some customization is typically included within the higher subscription tiers, more elaborate customization requests may incur additional charges. These fees are variable and contingent upon the complexity of the customization.
Integration costs
Integrating AI customer service software with existing business systems (e.g., CRM, ERP) may necessitate additional financial outlay, especially if the integration is intricate or legacy systems require significant modifications.
Training and support fees
Comprehensive training for staff and ongoing technical support may not be comprehensively covered under the subscription tiers. Businesses should inquire about these costs to ensure proper budgeting.
Overage fees
Specific pricing models may include limitations on the number of interactions or transactions the AI system processes. Exceeding these limits could result in overage charges, a critical consideration for businesses expecting high volumes of customer interactions.
Training process for implementing AI customer support systems
In today's fast-paced business landscape, customer support services are rapidly being transformed by artificial intelligence. AI-driven customer support systems enhance operational efficiency and offer customers a personalized and swift resolution to their queries.
However, the success of AI in customer support hinges mainly on the effectiveness of the training it receives. This instructional post is tailored to those integrating AI into customer support operations. From the initial onboarding of AI systems to ongoing training and continuous improvement, this guide provides insights to ensure a smooth and productive transition.
Understanding the basics of AI training
You need a solid grasp of the basics before trainingyou can train an AI system to operate within your customer support framework. AI training typically involves using large data sets to teach the system to recognize patterns and respond to input. The stages of this training include data collection, labeling, feature selection, and model training.
Data collection
Your first step is to gather a diverse range of customer queries and responses. The more varied your data set, the better equipped your AI system will be to handle incoming customer requests well.
Labeling
Once you have your data, it must be labeled. Labels can include issue categories, sentiment, or any other relevant information the AI needs to recognize and respond to.
Feature selection
Training an AI involves reducing your data to the most essential features to train the system more effectively. This includes the initial text, language, tone, and context.
Model training
This is the heart of AI training. Using labeled data, you teach the AI what outcomes you expect when specific inputs are given. This training continues until the desired accuracy is achieved.
Onboarding your AI system
The onboarding process is where you set up your AI system for initial deployment. It's crucial here to convey the specific requirements and business logic unique to your organization.
Data integration
Ensure that the AI has access to the correct data. Training data should mirror the live environment as closely as possible for accurate predictions.
Define objectives
What are the main goals of your AI system in customer support? Define key performance indicators (KPIs) and ensure the system's training directly aligns with these objectives.
Test for robustness
Before going live, subject your AI to various test queries to gauge its ability to handle real-world uncertainties and anomalies.
Ongoing training and model improvement
For AI toAI is not static. R remainTo remain effective,, it requires, ongoing training and improvement are required. Simulating the live environment and feeding new data into the AI system helpshelp it to adapt and evolve.
Continuous learning
Set up mechanisms that allow your AI to learn from every customer interaction. What it learns today might become outdated tomorrow, so continuous learning is non-negotiable.
Automatic model updates
Implement automatic update mechanisms to refresh your AI model with the latest training data and new features without manual intervention.
Performance evaluation
Regularly evaluate the AI’s performance against your KPIs. This ongoing assessment helps identify areas for improvement and inform your training strategies.
Advanced training techniques
Consider deploying more advanced training techniques toTo take your AI customer support team from good to great, consider deploying more advanced training techniques.
Reinforcement learning
Reinforcement learning allows your AI to learn from trial and error—receiving positive feedback for correct actions and negative for incorrect ones. This method can be an effective way to train more complex decision-making AI models.
Transfer learning
If you have multiple AI systems, transfer learning allows you to train a new model quickly by transferring knowledge from an older model. It's like the AI version of "building on what we know."
Human-in-the-loop
Including humans in the training loop can ensure higher quality. For instance, having your AI suggest responses that agents can verify and fine-tune adds a layer of curation that AI alone might not achieve.
Best practices for AI training
Finally, adhere to these best practices to avoid common pitfalls and setbacks during the AI training process:
Quality over quantity
Ensure that the data you use for training is clean, accurate, and representative of all possible customer scenarios.
Ethical considerations
AI training must also respect ethical and privacy considerations. Organize and store data in compliance with regulations like GDPR.
Skilled teams
A skilled team is critical for the success of your AI project. Invest in training for your AI trainers and developers.
Documentation
Proper documentation of your training methods and data sets is indispensable. This will simplify updating and auditing your AI system.
Agility
Be prepared to adapt and change your training methodologies as you learn more about your AI system and as customer needs evolve.
Following these detailed guidelines ensures that your AI customer support system is trained to its full potential and ready to deliver exceptional service.
Remember, the goal is not to replace human support but to enhance it, creating a more efficient and satisfying experience for your customers and support agents.
The collaboration between human agents and AI systems
When it comes to customer service, response time is of the essence. However, ensuring swift and accurate responses 24/7 can be a challenge that AI-driven solutions have expertly risen to meet. However, it is in attuned collaboration with human agents that this efficiency is brought to life. Integrating AI into human service teams was met with skepticism and apprehension in the not-so-distant past.
LiveCchat and CchatBbot integration
LiveChat has become an essential tool for businesses of all sizes. It allows businesses and brands to communicate with customers in real -time, directly on their website or mobile app.
Benefits of LiveChat:
- Improved customer satisfaction
- Increased sales and conversions
- Reduced costs
- Increased website engagement
- Valuable customer insights
ChatBot can answer customer questions, resolve customer issues, and generate leads. The software can also be integrated to increase efficiency.
You may consider LiveChat if you want to:
- Use pre-set messages to engage people automatically, or start chats with selected visitors when they land on your website and start selling from day one.
- Help customers across several communication channels by integrating LiveChat with WhatsApp Business, Messenger, Apple Messages for Business, and more.
- Connect LiveChat with leading e-commerce platforms to send in-chat product recommendations.
- Reduce tedious tasks with automation — reply twice as fast with AI Assist, cut reading time with Chat Summary, and get instant reply suggestions.
Get a glimpse into the future of business communication with digital natives.
Get the FREE report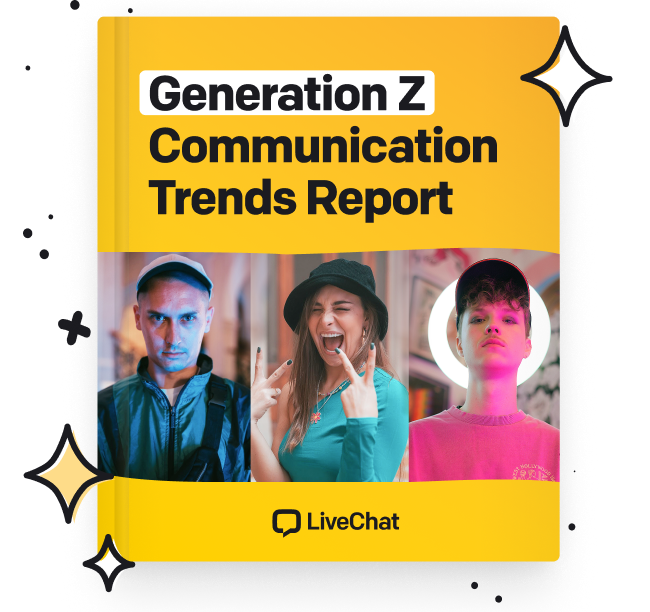
Comments